What is Multi-Head Attention (MHA)
What’s Multi-Head Attention (MHA)
In last post I have explained how the self-attention mechanism works. Today let’s take a step further and explore multi-head attention (MHA), which is the full version of self-attention as described in the original paper1. Since I have covered most of the foundation concepts in last post, this post will be short. :)
Previously, we mentioned that the self-attention mechanism has three import matrices.
$$ \mathbf Q,\mathbf K,\mathbf V\in\mathcal{R}^{n\times d} $$
Let’s assume we want to use $n_h$ heads. The core idea of MHA is to split the query/key/value vector into $n_h$ parts, each of length $d_h=d/n_h$. The transformation changes the shape of the matrices to:
$$ \mathbf Q,\mathbf K,\mathbf V\in\mathcal{R}^{n_h\times n\times d_h} $$
Note that the $n_h$ is put in the first position of dimensions.
What are the benefits of introducing multi-head? In my opinion, the key to understanding MHA lies in analyzing matrix multiplication and observing how the shape changes. Before applying MHA, the attention score matrix is computed as
$$ \begin{split} \mathbf Q\in\mathcal{R}^{n\times d}\\ \mathbf K^T\in\mathcal{R}^{d\times n}\\ \mathbf Q\mathbf K^T\in\mathcal R^{n\times n} \end{split} $$
So we get an attention score matrix here.
After applying MHA, the equations become
$$ \begin{split} \mathbf Q\in\mathcal{R}^{n_h\times n\times d_h}\\ \mathbf K^T\in\mathcal{R}^{n_h\times d_h\times n}\\ \mathbf Q\mathbf K^T\in\mathcal R^{n_h\times n\times n} \end{split} $$
As a result, we obtain $n_h$ attention score matrices. To provide some intuition, I have drawn a diagram (assuming $n_h=4$). The computation occurs within the corresponding matrices of the same color, producing multiple attention score matrices.
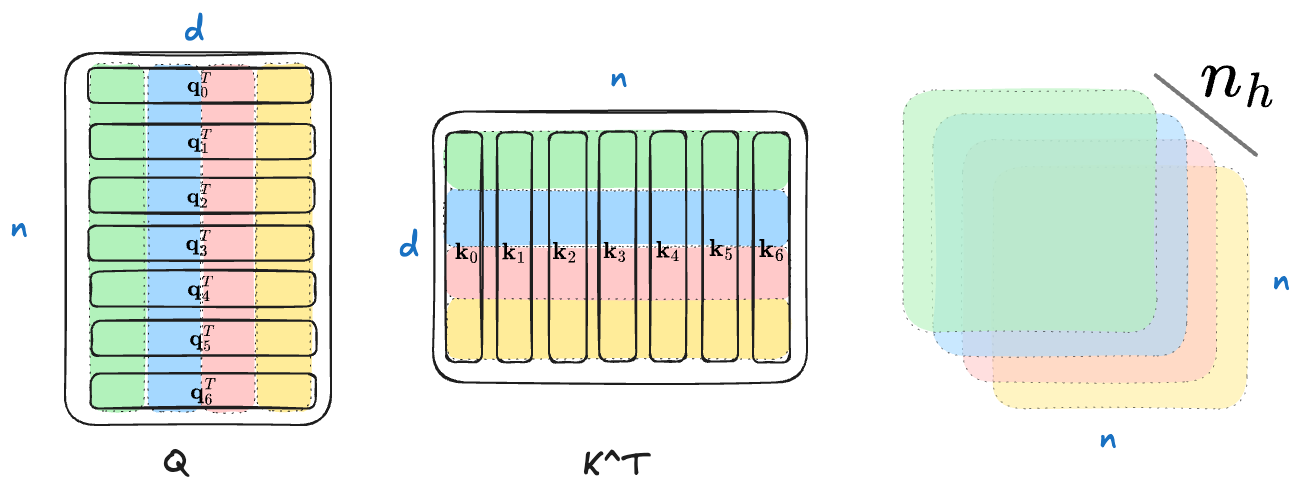
For simplicity, let’s ignore the scaling factor $1/\sqrt d_h$ for now, then the final output of MHA is
$$ \texttt{softmax}(\mathbf Q\mathbf K^T)\mathbf V\in\mathcal R^{n_h\times n\times d_h} $$
Finally, we can reconstruct the output by reshaping ($\mathcal R^{n_h\times n\times d_h}\rightarrow\mathcal{R}^{n\times d}$).
Implementation
The implementation is straightforward, building upon the self-attention code from my last post. The key modifications are
- The target shape of
bias
is changed to(1, 1, block_size, block_size)
. - The
q, k, v
matrices need to be reshaped. contiguous
should be called before reshaping forattn @ v
.
The complete code is shown below.
class MultiHeadAttn(nn.Module):
def __init__(self, n_embd: int, block_size: int, n_heads: int):
super().__init__()
self.attn = nn.Linear(n_embd, 3 * n_embd)
self.n_embd = n_embd
self.n_heads = n_heads
assert self.n_embd % self.n_heads == 0
self.register_buffer(
"bias",
torch.tril(torch.ones(block_size, block_size)).view(
1,
1,
block_size,
block_size,
),
)
def forward(self, x):
B, T, C = x.size() # (batch_size, seq_len, n_embd)
qkv = self.attn(x) # qkv: (batch_size, seq_len, 3 * n_embd)
q, k, v = qkv.split(self.n_embd, dim=2) # split in n_embd dimension
q = q.view(B, T, self.n_heads, C // self.n_heads).transpose(
1, 2
) # q: (batch_size, n_heads, seq_len, n_embd)
k = k.view(B, T, self.n_heads, C // self.n_heads).transpose(
1, 2
) # k: (batch_size, n_heads, seq_len, n_embd)
v = v.view(B, T, self.n_heads, C // self.n_heads).transpose(
1, 2
) # v: (batch_size, n_heads, seq_len, n_embd)
attn = (q @ k.transpose(-2, -1)) * (
1.0 / math.sqrt(k.size(-1))
) # attn: (batch_size, n_heads, seq_len, seq_len)
attn = attn.masked_fill(self.bias[:, :, :T, :T] == 0, -float("inf"))
attn = F.softmax(attn, dim=-1)
out = (attn @ v).transpose(1, 2).contiguous().view(B, T, C)
return out
Wrap-up
To summarize, the essence of MHA lies in the dimensionality. With MHA, although the query/key/value vectors are shortened ($d\rightarrow d_h$), we obtain more attention score matrices ($1\rightarrow n_h$). If each attention score matrix captures a distinct pattern, then MHA effectively captures multiple patterns, leading to improved performance :)